

For instance, when you want to remove numbers but not dates. There are cases where you might want to remove digits instead of any number. But don't remove this one H2O"Ĭlean_text = re.sub(r"\b+\b\s*", "", sample_text) You can use a regular expression for that: import re In some cases, you might want to remove numbers from text, when you don't feel they're very informative. "Yes, you got it right!\n This one too\n" "This TEXT needs \t\t\tsome cleaning!!!.", Take a look at the example below: import re If you're using pandas, you can apply that function to a specific column using the. Then, you can use that function for pre-processing or tokenizing text. I'd recommend you combine the snippets you need into a function.
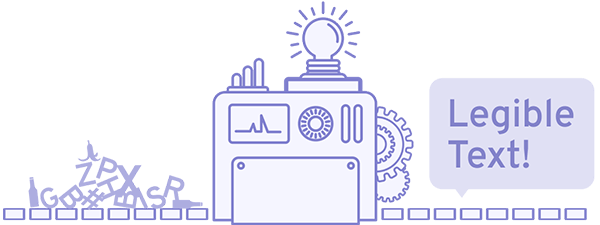
Then, you can check the snippets on your own and take the ones you need.
#Nltk clean text how to
In the next section, you can see an example of how to use the code snippets.

They're based on a mix of Stack Overflow answers, books, and my experience. I'll continue adding new ones whenever I find something useful. This article contains 20 code snippets you can use to clean and tokenize text using Python. Cleaning and tokenizing text (this article).I'm starting with Natural Language Processing (NLP) because I've been involved in several projects in that area in the last few years.įor now, I'm planning on compiling code snippets and recipes for the following tasks: So, finally, I've decided to compile snippets and small recipes for frequent tasks. At this point, I don't know how many times I've googled for a variant of "remove extra spaces in a string using Python." I end up copying code from old projects, looking for the same questions in Stack Overflow, or reviewing the same Kaggle notebooks for the hundredth time. Remove all special characters and punctuationĮvery time I start a new project, I promise to save the most useful code snippets for the future, but I never do.
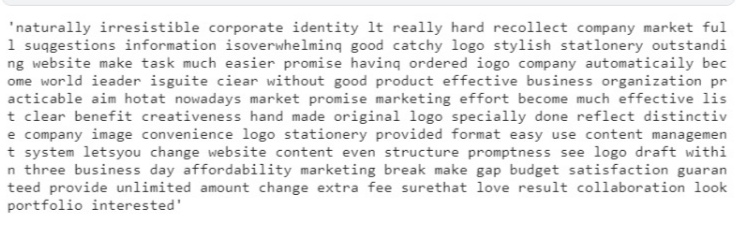
#Nltk clean text install
It is recommeded to install an optimized version of both. See here for more info: īecause clean_text is based on nltk, you need install blas and lapack libraries. Then setup the environment and indicate to distribute to build numpy with the openblas library. First you need get the code and compile it as usually. It is pretty easy to build an own optimized version of openBLAS. Remove stopwords and perform stemming Installation
